The Risks and Rewards of AI in Business Automation
- 0.5
- 1
- 1.25
- 1.5
- 1.75
- 2
Chris Clarke: Hi, welcome to GRC and Me, a podcast where we interview governance, risk and compliance thought leaders on hot topics, industry specific challenges and trends to learn more about their methods, solutions, and outlooks in the space. And hopefully have a little fun doing it. I'm your host, Chris Clarke. With me is Dorian Cougias, cofounder of Unified Compliance and the primary architect of the Unified Compliance framework in the compliance portal, the UCF Common Controls Hub. Dorian serves as an advisor and working group member to the Payment Card Industry Council, Financial Technology Forum, and other industry organizations. Dorian, you mind telling us a little bit more about yourself?
Dorian Cougias: Oh boy. I don't know how much you want to know about me and you guys can find it all on my LinkedIn profile, it's D- C- O- U- G- I- A- S. I've been around this industry before this was gray and the stuff that's way down here, before gravity took it, was way up here. We've been doing this for quite a while and it's a neat industry and I work with a lot of really, really brilliant people and that's what you really need to know. The great part is that I'm not the smart guy here. Every time I go into a meeting, I'm the dumbest guy in the room, which is pretty cool.
Chris Clarke: Yeah, that's a great feeling. Do you mind talking a little bit about what UCF is and just like what some of the benefits of using it are?
Dorian Cougias: Sure. Unified Compliance Framework is a methodology for we do mapping for you, defensible mapping for you, that's the most important part. It was born out of the need that was expressed when the person from Washington DC called me up and said, " Hey, look, I've got a problem. I've got four authority documents, regulations, laws, standards," that's what we call authority documents, we group them all into one. And he said, " I've got four of them. One of them says, close the tap. One of them says, turn off the spigot. One of them says, shut the faucet. And the fourth one says, swing the tennis racket." I said, " Okay." He said, " Well, how do you prove when they're the same and when they're different?" And I said, "Well, it's common sense, right?" He said, " No, no, no, no, no. We got to do it in language. We've got to do it defensible. We've got to do it in a way that we can then expand on this." And it wasn't as easy to do as I thought it would be. And so we had to come up with a methodology for searching text and having similarity and more importantly, dissimilarity rules. That's what the Unified Compliance Framework is, it's the framework for saying when is this similar to this? And therefore, harmonizing or de- duplicating the rules. And then when is that different? And more importantly, so when you look at it, we break it down into predicates and subject, let's go back to Fraggle Rock. Let's get to the English part. What's a predicate? What's a subject? All that stuff with Sister Mary Knuckle Buster back in grade school. And so synonyms, antonyms, hypernyms, hyponyms, very, very similar. And by the way, people can't do this. You have to have computers to do this because you have to have rule systems and there's so much of it that comes up. You have to have rule systems to start reading this for you. So one set of rule systems broke it into is this a sentence? Let's tokenize it. And here's the first word you've got to know what's our corpora, okay? Because all of your sentences, all of the stuff you're reading, that becomes your corpora and you're going to have to manage that. You're going to see a lot of that word coming up lately. And Vidia just did a deal with one of your competitors where they're using large language models, that's another word. The large language model is the biggest corporas out there. We're talking a billion words. You're not going to have that many words in your compliance dictionary. But the first thing that they said was, " Hey, in this partnership we're going to use these large language models, but you're also going to have to train them with your corpora. So right now, let's just say our corpora is four sentences. Shut the tap, turn off the spigot, close the faucet, swings the tennis racket. So the predicates have to go in there. And when you're using machines to read that, turn off is what's called a multi- word expression. So we had to build our machine system to look at multi- word expressions and be able to tag those. That's part of the framework. And then the other part of the framework is deciding when turnoff, shut and close are the same and when they're different, faucet, spigot, the other. And that has to tie a dictionary in. So that's the second thing you're going to need to know is how is your dictionary used to tie those things together? So really, the Unified Compliance Framework is a fancy way of saying, we can take all these sentences and put them in your bucket. And then we could take all of these words and when Joe was saying shut and Tammy's saying, turn off, and Fred over there is saying close, we know it's the same thing. So that's our dictionary part. And then the framework for harmonizing it is, we know that shut, turn off, close, spigot, faucet are the same. And then we, more importantly, know that swing and tennis rocket are not. That's really the nuts and bolts of the entire Unified Compliance Framework. It can come down to those simple things. How do you take the sentences, stick them in a bucket for examining it? How do you take the words, define the meanings, and then use those definitions to say, when is something the same and when isn't it?
Chris Clarke: And then allow Joe and Tammy to go and compare against that to see where-
Dorian Cougias: Exactly, Joe and Tammy and Sammy. And then taking it even farther and saying, okay, well if we're going to teach somebody to turn something off, there's this dude named Bloom, the Bloom's taxonomic rules. Matter of fact, if you're into education, this would be the book right here, I'll stick it up really close to the camera. It's about Bloom's taxonomy and you're going to hear a lot about it, especially with the cybersecurity communities and this NICE and the government's own a training curriculum. Because this dude named Bloom said, " Every time you go to do something, there's a level of understanding you have to do it." The level for turning something off is pretty short, but the level for maybe configuring, if you're configuring a router, well, there's a level of education you're going to have to have for configuring, predicate, this asset. And that has to match education levels. That's also built into our framework. The other thing built into our framework is that thing, you're doing something to, that subject, the light, the spigot, the tennis racket. When you start getting into compliance things like configuration guidance, you need to know what those are because I need to pass through a logic gate. Hey, when we're going to go implement this, you've got to go find that spigot, that faucet, that tap, and ensure that it's what? Turned off. So it sets up how you can audit something. And if anybody's ever seen My Cousin Vinny, remember My Cousin Vinny?
Chris Clarke: Yep.
Dorian Cougias: Well, it's cool because that's I was taught, funny enough, by a lawyer, this is how we have to audit things in compliance. My Cousin Vinny won his court case when, remember at the very end we're talking about he was cross- examining his fiance and she talked about the differential axle thing. Well, it was based upon can you examine this asset? Does this asset have this configuration item? Does it have a straight axle or does it have that differential axle? That's the same thing in audit for us in compliance. You have to look at the asset to know what you can do with it. When he was talking to the dude about cooking the grits, right?
Chris Clarke: Yes, 20 minutes.
Dorian Cougias: What was he doing? He was testing a process. When he had all those pictures in front of the guy about looking through that dirty window, through those bushes, through those trees, he was examining evidence. Same thing in compliance. You can examine records. You can't test a record, but you can examine a record. If you've got processes out there, somebody's got to observe the process. If you have an asset, you check the configuration for the assets. And that all goes into the compliance framework. And it gives us those rules for how to look at those subjects and predicates, what we can do with them and then how we can audit them.
Chris Clarke: That's so interesting. I love the example, and I think it's a nice segue. So you mentioned corpus, you mentioned large language models, and then in particular, I think the interesting part is defining that corpus as well. We encounter that decent amount here at logic gate where we call use cases within our platform applications, and that works if you know logic gate. However, if you're a typical, you work in IT and you say an application that would typically define logic gate as a whole, because that is the software application more broadly that is being defined. And so defining those at each level, and as it's relevant, it seems strong. As we start to go deeper into this, into artificial intelligence and a brand new framework. I know corpus large language model, those were, corpora, were new words to me. What are some other terms or just phrases that people listening to this or thinking about AI should be aware of?
Dorian Cougias: Okay, everybody who's listening to this podcast, this is how you show up your bosses. So there are 15 words you can use to show up your boss about artificial intelligence and how it applies to GRC that you've got to know. None of these are going to get you a date in the bar unless you're at some nerd bar.
Chris Clarke: That's what you think.
Dorian Cougias: Well, all right, if you're in Silicon Valley, it'll get you a date. I live in Las Vegas. My wife was reading what I was writing it, and I'm looking off to the left over here because I looked at it myself. Robotic process automation, my wife went, " Really?" But there are 15 core terms that you have to know, that apply to artificial intelligence as it applies to governance, risk and compliance. And funny enough, robotic process automation is one of the keys because AI in the GRC space is divided into three parts. It's divided into robotic process automation, one big chunk. It's divided into data and risk analytics that also can be used for harmonization. And then it's divided into this new thing that everybody's all hot and bothered about, but they have no concept of what it's really doing, it's going to be generative content. Now that's your ChatGPT, that's your other thing that's going on out there. So that's the world that we live in right now. Those three parts and robotic process automation is one of the key terms you need to know. And also about a third of how AI applies to what we're doing. And all of that, if you want to throw a term around to your board or your supervisors, it all falls under wrench tech. Yeah, we do AI wrench tech here.
Chris Clarke: I think a lot of those are new, but when you talk about, and you bring in robotic process automation algorithms, that's a little bit more familiar to me. But to your point, everyone's on this ChatGPT train, that's the hot new thing. I know the other two have been around for a while. Why do you think the ChatGPT has made waves in the more popular media in a way that algorithms and robotic process automation hasn't recently? Is it because it's just new? Is it because, you know...
Dorian Cougias: No, when data and risk analytics came out, it was new, but nobody gave a crap. I know, I was keynoting a seminar and I had 100 people in it and the other guys had 400 people. I was like, yeah, okay. I'm preaching to the nerds. The reason ChatGPT, and I was talking to the oldest son, who was just finishing law school and he's talking to me about ChatGPT like artificial intelligence is brand new. Wow, this is great. And I said, " Why do you think it's so hot in law school right now?" He's down at Chapman? And he said, " Oh, that's simple, because it writes for us. It thinks for us." So it's not thinking for you, it's really just regurgitating other stuff that's out there. And his answer was, " Yeah, but that means I don't have to." Risk and data analytics, you still have to do something with that. You still have to look at it, you have to apply it. Robotics process automation, man, you're deep in the woods with rule sets and everything else like that. That's for the seriously nerdy folks in the group. But anybody can use generative content to create my slides for me, create a tweet about this, write a silly poem. I was talking to one of the guys from Tootella, one of your integrators and I said, " What do you think?" There's a conference in town, they're all in town. And some of our clients in there, I went to speak and I said, " Well, what are you using it for?" And the guy said, " I wrote a children's book with it."
Chris Clarke: Wow.
Dorian Cougias: I said, " Is it any good?" He goes, " Yeah, my three- year- old thinks so."
Chris Clarke: That's awesome. It's so funny that you bring that up. This is a kind of side topic, but I was looking through stuff on this and I saw a meme around how we all thought when AI came, it was coming for our jobs, for doing these things. And what it really came for, it came for our art. It started to generate images. It started to generate poems and the things that we almost thought were like only humans could create. And so it's interesting how wrong we were in that prediction, at least-
Dorian Cougias: Well, when you really think about it's not generating, so there's a fine line between stealing wholeheartedly, which is what it does, and creating something absolutely new. Now that's the Skynet of the world. That's when it's, oh my God, they might come for us, when that thing goes live. Because right now, even if you look at the art they're using, and matter of fact, Italy is really off about this. The art that they're using to create new art will sometimes still even have the original artist's signature on the piece that it's copying out. So again, I gave you guys a couple of papers, one of the papers that people here are going to need to know about are the data protections and licensing affecting text for data mining? Because that's what these things do, they data mine. If you go and ask ChatGPT, " What are the core tenets of a Unified Compliance Framework?" It's literally going to steal the four paragraphs that I wrote, because that's the only thing in its corpora. And it's going to reword it slightly. I was kidding around with my lawyer. I said, " Look, if I took it in, who can I sue for intellectual property theft? Because that's mine and it didn't cite me." And that's the big thing. And ChatGPT right now, even if you ask it to cite somebody, it's just making crap up. It literally is. What was it? We did a test, a friend in Italy wrote a couple of papers and we knew the 10 papers that surrounded what we were writing because it was very, very esoteric. So we asked it to give us some content on the subject and then asked it, " Using Chicago manual seventh, whatever, give us paragraph and section on this and all the citations." And it gave us these ResearchGate citations, which we knew ResearchGate had. That's where they published the peer review journal that I write for. That's where they'd put it up there. And I thought, wow, cool. It's got ResearchGate citations. Then I went to look them up. Not only did they didn't exist, but after I got ahold of the editor of ResearchGate, they never existed, those titles, because he's got, he can go into his way back machine and his archives. He could see anything that's ever been published there. Not only were those URLs the wrong URLs, but the titles of the paper they gave had never been submitted. And some of the authors were up there, but a lot of the authors were grouped together in groupings that never worked together before. And then when I asked a friend of mine over at OpenAI, funny, they wanted to tap me as the head of governance risk and compliance at OpenAI, I went, " Oh, nope, not going to happen." And other than the fact that I'm like the CEO of a company, but I said to him, I said, " You guys have got a problem because you're making stuff up." And he said, " Well, it's trained on a large language model and it's trained on how humans write and how humans think and humans lie. And so if we trained it on that, it's going to do the same thing humans do. So why do you think it's any different?" There's no ethos, there's no morality to a machine. It will do the most expedient thing.
Chris Clarke: That's crazy. Yeah, I know there's been a lot of discussions around how to train it on the ethics of it and all that. So it's fascinating that the first ethical dilemma that it immediately does is lying. It's just not telling the truth around it.
Dorian Cougias: Right, and then when you catch it on it, oh my God, it's like I have a five- year- old nephew and I caught him fibbing the other day and I said, " I know you're fibbing." And he kind of gave a little bit and I said, " Oh no, come on, let's go." And then he gave me, and he finally fessed up to the whole thing. If you go to ChatGPT and say, " No, that citation's wrong." It'll say, " I'm sorry, and then I'll try to correct myself." And you say, " No, that's wrong too." " Oh, I'm sorry." And it will maybe try to work its way in and then finally it'll say, " I don't know where that citation came from." Even though it is does because it should be tracked in its corpora. So that's the other thing, when you're building a corpora in your corporations to train the model for what you're doing, you need to build your corpora management system so that as you're putting all those sentences in, you're tying them to the internal documents that they came from. Because the other big part that you've got to know, that you have to tie to AI, you actually have to tie to everything in compliance is the concept of explainability. And there's an explainability AI model that people can pull from, that you need to apply to these things. But everything you're doing has to have explainability. That's one of the things we built into the Unified Compliance Framework. If anybody ever goes to our ucfmapper. com, they can go up there and see how we're mapping things and they can even go and see how we're arguing with each other or the auditors about how some things are mapped because we have to do it to PCAOB standards. So they're going to have to build explainability into everything that they're doing with this.
Chris Clarke: It's fascinating you bring up the explainability piece because if we think about our typical use cases or everything we do in GRC, like if you do an audit and you're testing the control, you need to provide evidence on that the control has been performed. If you are performing a third party risk assessment and the third party says, " Yeah, we're SOC 2 certified." You never would just accept that. You'd be like, okay, it can be inaudible. And so it's fascinating that we as humans just inherently trust that ChatGPT is telling us something based on inaudible rather than pushing for that evidential collection off of it, especially in the GRC space where we are trained to want that documentation and that evidence as well.
Dorian Cougias: And so when you're looking at the generative AI part in organizations, because that's where everybody's going to want to play right now. Write me a policy based on this thing. Here's the challenge that I have for them right now, take one citation from an authority document and you have to follow, find a simple one. Let's take 100AC3, whatever it is, find one citation and say, " Write a policy statement that follows this citation," and watch. Then go on in and compare what you're reading with what it writes and ask yourself, could you defend that? You're going to find more than 50% of the time, you can't. I did this, I wrote a piece, it's up on our website somewhere about how it came out in the last newsletter. Matter of fact, when we were promoting logic gate, and one of the things was how to use ChatGPT to start writing policies and then how to check it for when it goes wrong and take those parts out. But what it is good at is generating RACI charts, who's responsible, who's accountable, who should be communicated with, who should be informed or maybe misinformed as the case may be. And then it'll do that and what really, really cool and I'm just so into, which part of compliance is code is it will then give a policy, if you feed it a policy, if you feed a procedure, excuse me. If you can then say, " Create a plant UML session flow diagram or user flow diagram or a deployment diagram based on this procedure." And it will create you an auditable diagram in plant UML right then and there. And if you look at it, they match and they've matched 100% of the time and everything we've thrown at it. So much so that we're seeing and plant UL is another one of those. So plant UML, this is fun, and so this is where that robotics part, that's the first piece and then that analytic part are starting to blend because now my organization, we're completely remote. I'm in Denmark, I'm in the US, all over the US. I'm in the Canary Islands. I want to make that my next office. We're everywhere. And when we have to go past our audits, we use the C4/ plant UML method to have our systems document where we live, because there ain't going to be a human in my organization that's going to keep up with where we're deploying parts of what we're doing. So that the robotics automation part is going out to Amazon and Microsoft and saying, document thyself using the C4 plant UML methodology. Deliver that documentation to us, so that we can then put that into our compliance portfolio. So the UCF is now accepting plant UML as one of the elements within UCF. And that is both then robotic automation of documentation and analytics because we can pass that analytics over to our cloud strike or something else like that, that says, " Okay, now examine this amorphous border and tell us what the risks and threats are that are going to hit that." Two sections of AI. And it would be really, really cool if I can get that generative part that says, " And then write the board report for me every month."
Chris Clarke: Right, yeah. That's always our dream. That's awesome. So we talk about those three and we talk about combinations of those three. When you think about the future and as we're going to continue to invest and grow on AI, which of those three keeps you up at night? Where are you worried the most about from an AI perspective and why?
Dorian Cougias: I'm not worried for me, I'm worried for your clients. And I'm going to go back to the two core things because Vince Lombardi, when he took over the Packers, famously walked in and said, " Gentlemen, this is a football," and started there. Everybody's into the surface level BS of using ChatGPT to write things and all this other crap. What they don't understand is you have to train the model, okay? That's what keeps me up at night because they're not training the model on what they're doing with their language. And what's keeping me up at night is we don't have a standardized industry methodology for establishing and maintaining corpora, that bucket of where we're going to put things. All right, you go to any organization, hey, listeners, go to your CTO, go to your CIO and say, " Hey, if we're going to do this thing, what's the corpora management tool we're using?" Yeah, crickets farting, that's what you're going to get. You're going to get crickets farting, okay? You got to figure that out. We can help. We maintain a corpora. I don't even have a corpora service or API to sell you. I'd love to say, " Oh, inaudible I have the answer here." I have one for me, which is very, very, very robust, but I don't have one for you. And you're going to have to build one. And nobody's talking about an industry standard for that yet.
Chris Clarke: So can I pause you real quick on that?
Dorian Cougias: Yeah, yeah, yeah.
Chris Clarke: And we're talking about that through the lens of ai. You need some common definitions, you need some common corpora. Why is that different than a human writing or hiring someone to write your policies as a human versus going to ChatGPT? Where does-
Dorian Cougias: Whoa, okay.
Chris Clarke: Why is that different?
Dorian Cougias: I'm going to give you a real for instance. Much apologies to you, Mr. Jackson. I'm now going to tell a story out of school. Michael Jackson is, believe it or not, his real name was Michael Jackson, and I loved him. He worked for the FDIC and the FFIEC. And by the way, he's like 6'4", and he hated it when you would walk up and go, " I like Michael." But they came to us, one of our earliest clients, and they said, " We got a problem. Humans are writing our FFIEC audit guides." I said, " Okay, what's the problem?" He said, " We got nine of them." I said, " Yeah." He said, "Do you know how many different ways we're saying the same thing and using the same words to say different things?" When we did de- duplication and harmonization of their internal documents, not industry documents. Look, here's one document, this one document, each document had anywhere between 40 and 60% overlap because they were saying the same thing different ways or different things the same way. So when you have multiple humans working on, this is why I say humans can't do mapping. These are not stupid people. These are really smart people that I really, really, really respected and respect to this day. I make fun of Michael because he became a friend and I can and I'm out of arm's length because he'd pound me for telling these stories. But seriously, they had 60% duplication. That's why you need a corpora. That's why you have to have, in this world, you have to have this thing to put it together and you need that second part, which is a corporate dictionary. Now here's the second story on this one, and Stephen Paliero can back me on it. Jody Mac from Moxy PLC was there, The PR Firm, a bunch of people from NIST, I know you NIST guys are going to yell at me when you hear this one. So I was really, really mad. We went to Gaithersburg, we met with the NIST guys and they were first coming out with the NIST cybersecurity standard, and they'd asked us to work on it and help de- dup it and map it. And I realized that they were talking about cybersecurity different throughout the document. They had multiple offers and typical Dorian fashion, I'm kind of like a bull in a China shop, if you haven't figured that part out yet. I got mad and I grabbed them all in the same room and I said, " Okay, this is horse crap. I'm reading this thing, and the way I'm reading it, I've got a bunch of different definitions of cybersecurity because you're using it in different ways." So I gave you everybody a piece of paper and a pen, and I said, " Without cheating, write your definition of cybersecurity." I won't tell you how many authors were in the room. I picked them up and there was one more definition than there were authors. And I said, " Okay, how does that work?"
Chris Clarke: I don't even know how that math works.
Dorian Cougias: I said, " How's that math work? Did somebody spawn another invisible person here? How did we get more definitions?" And then we started looking through them and it was just slightly less than the total number of people there materially different definitions. And I finally said, " Who wrote two?" And one guy raised his hand. I said, " Why'd you write two?" He said, " I didn't know which one was right." I said, " Dude, you're writing the National Cybersecurity Standard. How can you have two definitions? And how can this odd inaudible group of people come up with this many materially different definitions and not talk to each other?" Because that's what humans do. Anybody's ever been married, darn well knows that you use the same words mean different things. And different words mean same thing because you've had that conversation with your wife, your kids, everybody except your dog, because your dog intuitively knows what you're talking about, your cats are ignoring you, so they don't even come into the picture. The only ones that know what's going on really in the world is dogs, because I think dogs are just sucking it out of our brain, what we really mean. They're just not listening to the worst. But everybody else, we're doing those different things. Machines don't do that. And how these large language models work, how AI works, is it literally, I don't know if I sent the screenshot to you guys last night or not. But I grabbed the screenshot from the Amazon package we use, which is very, very advanced. And right in there it says, " Here's how to tie in your synonyms, your antonyms, your hypernyms, your hyponyms. And your dictionary will go into our reading of your corpora, so that our similarity engine can work." Because when that guy says privacy policy, and that person says personal privacy policy, and that one says the data privacy policy of personal information, we know it's the same thing. That's why the second part of that puzzle is your dictionary. And ain't no one talking about how to handle that, either of those two things.
Chris Clarke: So I'd be interested in, if you're looking at a risk management program, you're setting thresholds. So with a dictionary, and I'm going to just blame English, but with the English language, you're always going to have some level of interpretation and difference in defin... So where do you recommend organizations set their risk threshold around that corpora and around that dictionary? And is it okay to have a little bit of wiggle room on what cybersecurity means? On what-
Dorian Cougias: So if you're doing it right, I'm going to go back to that second part, the analysis and the harmonization. When you're sucking your data into your corpora, your NLP engine, natural language processing engine is going to do this thing called extraction and interpretation, and it's going to find multi- word expressions and you're going to have to teach it. And as you're doing that, you're going to be assigning, ah, this is the term, and this is the definition of the term in that instance, okay. This is the definition of the term in that instance, the definition of the term in that instance. The wiggle room comes down, the risk comes down the more you're using those definitions, because then it can look at the instances. It can say, " Okay, in that instance, we understand that that's the use. And in that instance, we understand that's the use." Does that make sense?
Chris Clarke: Yes, yeah. So you are training it in some way.
Dorian Cougias: You're training it on the definitions and what it's called for it. And if anybody really wants a term that's way out there, I didn't even put it into the terms you need to know, it's called definition and coded distance vectors. All of these guys do this stuff with vector analysis. It's drawing things and whatever. I have a hysterical paper I wrote on similarity. I started out, I say, look, anybody read Curious George, the yellow man? You got yellow man, you got a dog, you got a banana, all right. What are the similarities? Well, you could say the yellow man and the banana are both yellow. You could say the dog and the yellow man are living things, right?
Chris Clarke: Yeah.
Dorian Cougias: Can you have any similarities between a dog and a banana? When we gave this similarity test to a bunch of people who theoretically do mapping, and one of you guys works for Vanta, but I'm not going to name your name and you all sue me if you want, that guy doing the mapping for Vanta actually said, the dog and the banana are similar. And I said, how did you get... I wrote this up in a paper after that, I was so mad. I said, " How did you get there?" He said, " My dog likes bananas." That was his personal similarity engine. That would never fly with an auditor, that would never fly with linguistic anything. And so people work that way, computers don't. They use word vectors, they use sentences, they use definitions to create these relationships. So when you go to Google and you say, " I want to buy some apples," it will tell you where the grocery store is. But if you say, " I want to buy an Apple earbud," it's going to tell you where the Apple store is because of the context. We're still looking at the word apple, but apple is a different context.
Chris Clarke: That's a fascinating example.
Dorian Cougias: And by the way, that's a real world example.
Chris Clarke: The whole time I was trying to think of, well, what's the similarity between a dog and a banana? What am I missing here?
Dorian Cougias: There isn't one.
Chris Clarke: Okay. That makes you look-
Dorian Cougias: Literally, I had to go to our artist inaudible. We're putting this in a white paper. So we use that now to teach what computers do. So computers, you give them good rules. So that's the explainability part, okay. It's the rules. You can use the rules. You can take that to an ISO auditor, a PCI auditor, a PCAOB auditor. You could take that to AON Insurance. You could take that to Lloyd's and you could say, " This is the rule set we're using." Great thing about computers are they're not going to say the dog likes the banana, because that's not in the rule set. So given this rule set, given definition encoded distance vectors, and the fact that we trained it on our corpora, and we said this term with this definition and this context, this term and this definition and this context gives us contextual wiggle room, brings the risk way down way that we can use it and gives us explainability. But the risk is massively high if you're not using your own corpora because it's going to make stuff up. Excuse me, it's going to make stuff up. If you don't have that dictionary tied in, it's not going to know your contextual use. And again, it's going to make stuff up. So that's how you reduce the risk.
Chris Clarke: I guess the flip side of that question, so that's where the greatest risk is. Where's the greatest opportunity?
Dorian Cougias: Oh boy.
Chris Clarke: Or I guess where isn't the greatest opportunity?
Dorian Cougias: Well, the realistic greatest opportunity right now is still in harmonization and deduplication because it brings clarity. If you look at what some of these regulators write, their job is to obfuscate the simple by using the worst terms possible to mean everyday things. And some of the stuff I read, I told my wife, " If I ever come down with terminal cancer, I'm going to DC and I'm going to find some of the people who write these things and I'm going to start slapping pies in their face. I don't want to hurt anybody.
Chris Clarke: Bucket list for Dorian, go to DC with pie, right?
Dorian Cougias: That's right, that's right. If I'm dying, I'm going to DC with a whole bunch of whipped cream pies, and I'm starting smacking people till they throw me in the loony bin because they obfuscate this stuff. But if you use AI to harmonize and de- duplicate. First of all, the language is going to be a lot simpler because AI is going to look at the personal indemnification policy of the usage of private data and go, " Oh, that's a privacy policy." And that thing just shrunk to this. And they would say, " Must be read, interpreted, and put into account for..." In other words, don't screw it up. So harmonization, de- duplication, simplifies it. Tells the people at a 9th grade, 10th grade level what they need to do. Because trust me, I sit on a lot of boards, all my fellow board members, that's about their level of not education, but their level of listening to these things. I sat on a board meeting the other day and a dude was like this. And he's like, I was looking over and dude's watching TikTok. Like, " Yo, we're listening to a security briefing." He goes, " Yeah, I'm getting every fifth word." So that's the way you got to present compliance. That's the biggest win you can get right now. And if you're doing it with AI, the cool thing about AI, maybe we should throw another term in, it's called named entity recognition. I think I'm going to go and stick maybe a 16th or 17th term in here, named entity recognition. So what that is, is it's reading, let's go back to swing the tennis racket and turn off the faucet, spigot, whatever. It knows that those assets fall into classes. It knows that I should say those predicate, those subjects fall into classes. They can be assets that you have to configure. They can be processes you have to put into place. They can be records that you have to maintain and/ or fill out a certain way because they'll actually tell you, maintain this record and ensure this is in it, and then ensure this is in it. And so the AI will read that and then hand over to logic gate, " Hey, this sentence says this, and by the way, this, that you've got to do it to is now this thing that you better go find. That's going to be evidence later in an audit. And do you have that record? And you can pass that over to the records keeping people, and it has property, it has metadata properties. If PCI says, " Maintain the blah, blah, blah record," you got to know that somebody's got to implement that. You're going to have to put it in your records management policy. Pass that over to them. AI will tell you that. AI will tell you what to look for when auditing it, so you can pass that over to the audit. AI will do all of that while it's reading it and it will all of a sudden understand it, make it a name density, and then automatically say to logic gate, here's all the JSONLD you need to know about how to implement this and how to audit this. And people ain't going to do that. That's your biggest win right now is making it simpler. And then also at the same time, telling me how to implement it and then how to audit it.
Chris Clarke: Now I'm excited. You're telling me I can make it simpler for me to do my job and maybe what I need to go do, that's incredible.
Dorian Cougias: Once the word is in, once it's in the dictionary, our compliance dictionary that we have has about half a million compliance terms. And auditors and implementers already have said, " Yeah, here's the named entities and this is what you need to do to implement them and maintain them and audit against them." And that's already in there. And that the other cool part about AI and computer systems is once it's there, it's there. You don't have to think about it again. Computer reads at the speed of electrons. It sees that and says, " Yep, I could pass that off. Boom, got it, got it, got it." And then only comes up with the stuff it doesn't know.
Chris Clarke: So I didn't have any other questions directly or related. Is there any other last thoughts you'd like to leave?
Dorian Cougias: Yeah, there are actually a few. I took some thoughts on this. When GRC first started, and I was literally in the room when Mike Rasmussen came up with GRC, lest anybody argue, Mike Rasmussen coined the term. I know, I was there. I wish I had coined the term, but I didn't. Nobody had to think as much about implementation of GRC as they have to do today because of AI. If you're going to apply AI in GRC and you're going to have to apply AI in GRC, it's just the way it's going to go. It's not like we're going backwards, guys. Electricity's here to stay, if anybody's wondering and AI's here to stay, just like electricity, it ain't going to go away. We're not going to be lighting with gas. We're not going to be going back to manual mapping and making stuff up. That means that you are going to be working with internal corpora and external corpora. You're going to be working with intelligence machines that you don't own, with data that you don't own, plus data you do own. You're going to be teaching a machine that sits somewhere in some cloud owned by OpenAI, by Google, by Amazon. You think it's weird that Alexa is listening all the time. Now you're going to be giving it your policies, your procedures, your risks, your threats, your configuration guidance. So the very first thing you need to think through is who owns the data and what license model you want to use in order to apply AI at the GRC level, because there are a lot of license models out there. And you are going to have to come up with a TDM, text and data mining license that applies to your content that you want to use in this tool, because some of the shared learning is going to be shared with other people. What are you willing to sit into another model? What are you willing to be generated elsewhere? What are you willing to do whatever, and what must you keep secret? So that's the very first thing you have to think through before you think of your corpora, before you think of your dictionary, before you think of any of that. This is a new world where the license is affecting this and is everybody bought in on it? Because you're going to have to get them bought in on it. Does that make sense?
Chris Clarke: It does, yeah. We already have examples like Samsung's IP loss because they didn't protect it in some way. It's interesting to think about that through the lens of policies where you would expect, you can go to extremes with this, is either this data sharing will improve everyone's policy making-
Dorian Cougias: Rising tide lifts all boats.
Chris Clarke: Right, or the flip side is everyone's exposed because everyone knows everyone else's policies in some way. And so it's an interesting like how do you walk that line or which way will it go? Is there a momentum shift? So it's definitely something that I'm going to do a lot more research on and-
Dorian Cougias: So yeah, so step one is really how do you define a machine- readable friendly license in a cloud contribution environment, okay? Because there's this cloud content below everything, like the content that the UCF maps, so it's regulatory stuff. Sitting on top of that is your data that you might or might not be sharing with other people. Sitting on top of that is the generative stuff that's going to be created out there. Okay, what's the data license your organization is willing to live with for machine- readable environments? Because that's the environment you're coming into. It's all going to be compliance is code. In order to do continuous compliance, it's going to have to be compliance is code, machine- readable at some point. What are you willing to live with? And then before you even put your corpora in, how are you going to define and manage your dictionary? Because I was at RSA walking through, how many vendors were out there talking about, " We will go find your data and put it in," which means classification taxonomies, you're going to have taxonomic structures, classification structures, and data dictionaries. That's part of that dictionary I was talking about. And every last one of the guys I talked to. " How are you managing the data dictionary?" " Oh, we got our own." " How are you managing the..." " Oh, we got our own. We got our own." I said, "Do you guys ever talk to each other?" They don't even have the same structures. I got three of them to talk to me. I won't name you guys, I won't out you, but wow. They're very far from working with each other the right way. And so how then are you going to structure your dictionary? For the very first time at the end of this month, I'm going to Boulder and I'm presenting, I'm a member, we, my company, not I, it's not that the Royal I. We are members of the Dictionary Society because we maintain compliance dictionary and how far in back of this curve the dictionary guys are is I'm presenting for the first time a federated dictionary structure that I've got Oxford, Chicago, Prentice Hall, Miriam Webster, Wordnik and Free Dictionary, Cambridge is in there too, to partner with. And I'm showing them everything that's wrong with how we're doing this API driven structure to even work together. And we're the Dictionary Society. And if we don't get it together and we don't have a standard way of querying and have a standard JSON that encompasses data dictionaries, if we can't agree on it, how can vendor A or B or COVID, who don't do dictionaries for a living agree on it? We're not doing that until, I think my presentation is June 1 in Boulder. If anybody's at the University of Boulder on June 1, come and see me afterwards. I'm pretty sure I'm going to need a whiskey, I'll buy. And we're literally, right before I got on the call to tape with you guys, I was seeing for the very first time how we're adding content from search to dictionaries and finding it from all those guys and finding it out of the ECFRs and the governance people and applying it to a corporate glossary and how we're actually bringing it over for the first time with attribution and licensing, so that a person can have a corporate glossary. And that's in alpha, that meeting, demo sprint right there, that was a demo of a first sprint. If we're that far behind, hopefully by the middle of June we'll have a way of talking to logic gate about how you might want to apply a dictionary for this. Right after the meeting in January, I'm getting on an airplane from Denver flying straight to DC, talking to a whole bunch of multi- letter clients that are out there and telling them how to put their dictionaries in, based on what we all agreed to in Denver the week before. I will guarantee you I'll be writing that stuff up on the plane and telling our programmers what to put, and then there's going to have to go up to GRC schema to become part of schema. org and then get in. The industry ain't going to be ready till what? July, August, September. So plan now, but you have time because there isn't going to be real implementation till then. If somebody's telling you that the upside is, " Hey, you got some time and we're all talking about it and let's get it in and get it in right." The bad part is somebody's out there telling you they're doing a generative AI and they're pulling it in with their corpora. Yeah, they've got a bridge to sell you.
Chris Clarke: Yeah, that's fascinating. There's a lot in there, including just the dictionary summit to define everything and how that naturally, there's this time. But in that time, even as people are starting to get ahead of that, there's risk there of using definitions. But at the same point, how do you prepare so that when it is ready to go and take advantage of that?
Dorian Cougias: As long as you're telling your board, " Hey, there's a lot of risk there." I don't know if you guys have seen the new SEC rules, the SEC rules for broker- dealers in public companies say the board has to be aware of cybersecurity. The board has to be aware of continuous compliance. The board has to start putting its threat and risk reports in Inline XBRL. I got to tell you, like 20 people know what that means. We do. We sat on the committee, and it's one of the things that we're mapping in. But how many CIOs, CTOs, and CISOs know how to read a human version of a report, a risk and threat report, and then ensure that it's the exact same thing coming out in Inline XBRL? How many of them can do the human readability to machine- readability inaudible. Yeah. And funny, because if it was actually built right, using Markup and using Legal XML or a Akoma Ntoso or some of these things you guys have never heard of, we do a demo in- house where we could take the machine- readable one and say, " Here's the URL for the machine- readable one. It comes out in JSON and XBRL, and then you add. PDF and it goes in, it reads it, turns it into a human- readable format from the exact same document. Because you can embed all of that and then the humans can read and go, " Yes, that's what it says." Because even I can't read XBRL. And how many people have you even seen to be able to write that report and have it come out in machine and then change a part of the URL, so that it's literally the same evidence. But now with this thing on the end of it. HTML or. PDF or whatever, it now turns it into human- readable. You can do that, but I've seen four organizations to date, us included, actually that's three and three quarters with this finger, do it successfully.
Chris Clarke: That's fascinating, yeah. And just to confirm that, you're saying that today you all can define the machine readable into the human- readable, but the reverse of that is much more difficult and undefined.
Dorian Cougias: And we're going to ensure that we in logic gate don't make the same problem. One of our past GRC partners and the past for a reason. We do all this mapping and we did a whole bunch of mapping for one of the clients, all their policies, procedures, things like that, like million and a half dollars worth of mapping. They put it into the GRC tool, and when they went to edit it, the GRC tool stripped out all the mapping because it uses just simple RTF, which is the background, that's the foundation to work. And when you go into your whatever SaaS based system, you got that little ribbon, bold, metallic, all that stuff, it's either putting it into YAML or RTF or Markdown or Docx, something like that. But it's not tagging it like SGML or XBRL or OSCAL if you're doing NIST or Akoma Ntoso or Legal XML if you're doing international stuff. Those are all the structured frameworks for writing. And if you start out the human way, without doing it structured, if you start out in Word, if you start out just writing your policies and procedures in there, you ain't moving it over to a machine- based thing because there's no formatting under there that tells the machine this is what's going on. There's a really cool company, it's a really nice guy. I can't think of his name. I wanted to buy the company until I found out that everybody, he wrote these interpreters. But I said, " Wow, that's really cool. I want to buy you. I want to buy you, I want to put you on inaudible, because you have this interpreter and you know can interpret a word and you can put it into SGML or Legal XML... I could just buy you, put a plugin into everybody's writing a policy and procedure and I can bring it into the structured format." Because a lot of what the UCF does is take these documents and get them into a structured format in our corpora. We have millions of dollars and 255 patent claims on how to do that. This guy was doing it and I said, "Wow, that's a method I've not seen before. I'm going to buy the company and the IP." Until I found out he has to manually do it for every company, because there's no Word- based thing that says, when I write a policy or procedure, here's how we're going to structure it. So the best you could do is you could work with a GRC firm or a policy management company that's building structured policies, that's building structured documents to begin with, that's giving that machine the knowledge of what to do with this paragraph or that paragraph. NIST 853 took a very, very, very long time to restructure the writing of it so that it would come out in OSCAL, which is a structured vocabulary. So that you know that this paragraph is informational, that paragraph has a mandate. This paragraph talks about training, that paragraph talks about auditing. When terms come in, must, shall, may, oh shoot, I was just doing a paper. It's probably a paper in the back of this screen somewhere and I can't show you my board because it's too far away and I'm realizing looking in here, you couldn't see it anyway, of how we break down a sentence and say, the organization must do this before that. Well, the must is modal. Could also say shall, could also say may. Do, okay, well now here's this thing. It's got a Bloom taxonomy to it. To what? Is there a time differential? Is there a do this after that? So we're breaking it down into all of those things, NIST 853 and OSCAL is broken down into all of those things. You go tell me somebody's got a policy procedure manager today, or Microsoft Word document where they're going to go and tag all their texts and say, " This is modal." When it says have eight characters, upper and lowercase in the password, how many people are going to take the eight characters and say, " This is now an end stream variable, and end stream variable can be put in by the organization's standards." By the way, that's what NIST 853 does, because it brackets it. That's the same thing we do when we're interpreting this stuff. We're putting all these brackets in, we're putting all this NLP stuff in underneath it. It's easier start with structured text and do something with it than to write an interpreter to look at all the texts and examine it, because that's where the NLP engine comes in. That's where your corpora and all that local training comes in. Because we're basically training you to think like a machine, so the machine can understand it. I'll get shot for this. My wife is famous for go get the thing. " Hey, go get that thing. You know the thing, it's over there by that other thing." Yeah, yeah, right. Tell machine that, good luck. But we've been married long enough I actually get what the thing is by that other thing because I know where the other thing was-
Chris Clarke: With enough training, we know.
Dorian Cougias: With enough training, exactly. With enough training, inaudible what the thing is. In this context machine, the thing is the spatula.
Chris Clarke: Yeah, that's awesome. Well, I appreciate you sharing all that. I've learned an incredible amount today.
Dorian Cougias: Oh, good.
Chris Clarke: I appreciate so much. Love to end on a little bit of a fun note if that's all right.
Dorian Cougias: Sure.
Chris Clarke: We're trying out some of these new kind of like podcast segments. One of them is we're going to call risk or that. And so like a would you rather, so I love that you mentioned this. When you think about dystopian future AI, you prefer Terminator or 2001: A Space Odyssey?
Dorian Cougias: Oh, I would have to say I prefer Terminator because I never really understood the ending of Space Odyssey. I know where Terminator's going. I know how to deal with that.
Chris Clarke: That's fair, probably the same way. And I don't really get that-
Dorian Cougias: And I'm still scared of how.
Chris Clarke: As we all are.
Dorian Cougias: Yeah, yeah. Anytime I go, I have a friend, oh, I don't have one, he's got one of the big red glowing things in his man cave and anytime the thing lights up. I was like, " Yeah, okay. I'm done with the whiskey."
Chris Clarke: That's your cue to leave.
Dorian Cougias: That's my cue to leave. I think he does it on purpose.
Chris Clarke: Oh, he probably knows now.
Dorian Cougias: Yeah, I'm very Pavlovian that way.
Chris Clarke: Yeah, flip side of that, who would you rather have as a best friend, Data from Star Trek or C- 3PO from Star Wars?
Dorian Cougias: I'm going to give a nod to you John, I'm going to answer neither of the above, Q.
Chris Clarke: Okay.
Dorian Cougias: John de Lancie, because I know him. So yeah, if you guys go into the way back machine, type my name in, type Cbold, type John de Lancie, Leonard Nimoy, you'll see I launched a software company with these guys and then I had to go pay penance for doing that and go to a Star Trek convention. I met Brent Spiner, very, very, very nice man. But if I had to choose between the two, it would be C- 3PO because I could probably play more tricks behind his back than Data, because I don't think his head can go all the way around.
Chris Clarke: Yeah, yeah. I love that. Yeah, that's awesome.
Dorian Cougias: And he's very British in his mannerisms.
Chris Clarke: Very much so, that's awesome. And then last one, and this one's a little bit more actual risk, but autonomous AI driven cars or humans driving cars.
Dorian Cougias: Humans because there's explainability behind them and I don't understand the autonomous part of the cars yet. I know humans are going to do stupid stuff, sorry. I know humans are going to do, so when you splice this, I know humans are going to do stupid stuff and if they're starting to swerve or whatever, I could figure that out. But for a car to blindly do something that I wouldn't be able to anticipate, I don't know enough of the rules yet that an autonomous driving system would apply to be able to anticipate, while I'm human driving my car, it doing anything stupid.
Chris Clarke: I love that you say that. So I'm from Pittsburgh, Pennsylvania. I don't know if you know anything about it, but we are terrible drivers. Sorry entire-
Dorian Cougias: Is that the one with the river and the restaurant that goes up on the thing?
Chris Clarke: Yep, yep, exactly.
Dorian Cougias: Oh yeah, I've been there. Yeah, you guys can't drive.
Chris Clarke: So three rivers. So my brother- in- law works for Auton... I don't know if I'm allowed to say this either, but he works for AI, kind of autonomous driving vehicles car. And the reason they picked Pittsburgh is Pittsburgh has this rule, this unspoken rule called the Pittsburgh left. And it is where you let, so two directions left turn lane, the oncoming cars, if you have a green, you actually let the first car in the left turn, turn before you go. And so the thought is if AI can do it in Pittsburgh, it can do it anywhere.
Dorian Cougias: Wow.
Chris Clarke: And so that's where they've been testing a lot of autonomous cars because there's like Pittsburgh rules make no sense and they put fries on their sandwiches. And then that's how they start to test in the worst, riskiest scenario.
Dorian Cougias: Wow. I'm glad I've never driven, I've always taxied through because I just go into division.
Chris Clarke: Yeah, and most bridges in the US, I think.
Dorian Cougias: Yeah, there were a lot of bridges. And notice, you and I share a corpora because I said that thing going up to that place, and you went the funicular to the restaurant.
Chris Clarke: Yeah, the incline.
Dorian Cougias: The incline.
Chris Clarke: Big thing, yeah.
Dorian Cougias: And see that's another thing, funicular and incline, same thing, two words, same meaning. You'd have to have a dictionary if this was an internal thing for our corpora.
Chris Clarke: You're writing up a story about it, yeah.
Dorian Cougias: Yeah.
Chris Clarke: Awesome. Well, thanks for playing along with me on that one.
Dorian Cougias: Well, thank you.
Chris Clarke: That was fun.
Dorian Cougias: Well, I'd love to come back and I'd love to, in between maybe even get a couple of questions to answer from folks. Everybody out there, I don't want to scare you. It shouldn't be a scary thing, but you have to think through some things. And you have to bring your leaders in to let them understand the risk. If you're going to go forward, cool. Just tell them what the risk is and let them accept or not accept the risk. You're going to have to accept the risk who are using it right now and you know, because we're all telling you, it's going to lie. It's really not lying because it has no ethics. So it's going to do what it does. It's going to put content down that you asked it to put down. I thank you all for listening to the ramblings of a semi madman today and hopefully we got you thinking about some of this, because that's what you need to do.
Chris Clarke: You're selling yourself short there, Dorian. Thank you for coming on, this was incredibly exciting. I'm pumped with what we're going to go and learn more about. And honestly, the next reading for my next couple weeks is going to be on all this. And thank you for all of you to listening to another episode of GRC and Me. Been a blast.
Dorian Cougias: Thank you, everybody.
DESCRIPTION
In just a few months, artificial intelligence went from a fringe technology to full-speed ahead with the public release of ChatGPT. This fascinating technology has the potential to revolutionize how we automate our businesses, but there are numerous reasons to give pause before integrating it into your organization’s operations. On this episode of GRC & Me, Dorian Cougias, Co-Founder and CEO of United Compliance Framework and Chris Clarke sit down to discuss the risks and rewards of embracing AI-driven automation, corpora management, data ownership, and the necessity of double-checking everything generative AI spits out.
Today's Guests
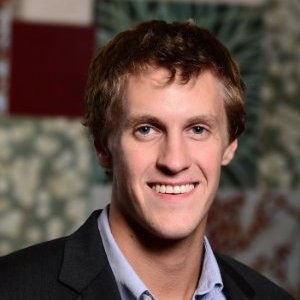
Chris Clarke (Host)
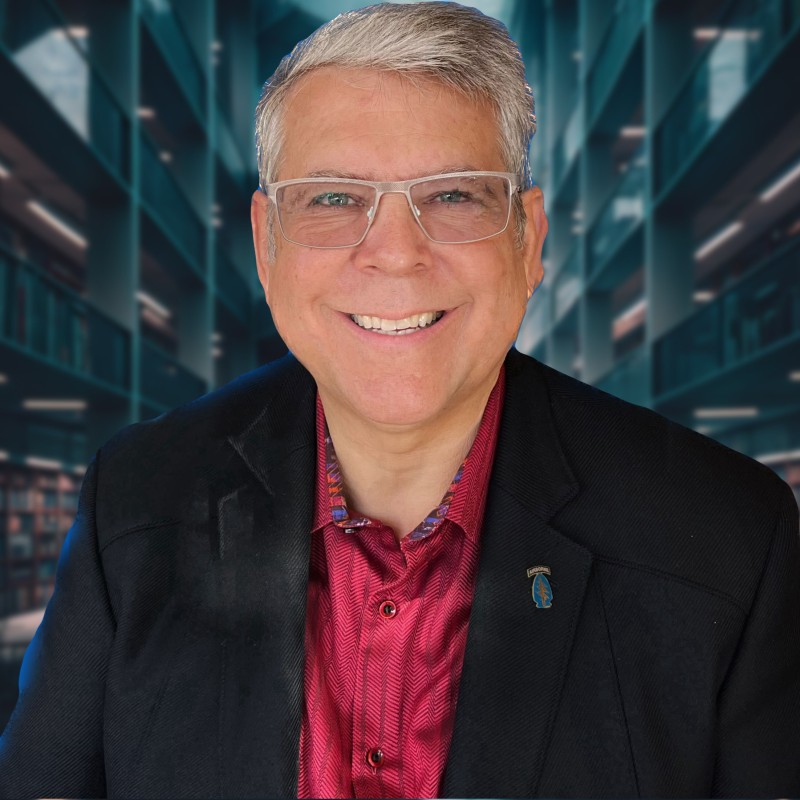